The Problem
Machine learning bias is dangerous, and fortunately, many in the AI community are working to redress these biases. But some of the algorithms developed to ensure AI fairness may in fact be more dangerous. These algorithms were built with very simple of definitions in fairness in mind, like reducing gaps in outcomes between different demographic groups. The result is that in attempting to close gaps, some algorithms may actually degrade performanoce for groups, with potentially devastating consequences. (For instance, algorithms may downgrade a formerly "high risk" cancer patient to "low risk" to equalize outcomes across groups.) The result? "Fairness"...that is actually quite unfair.
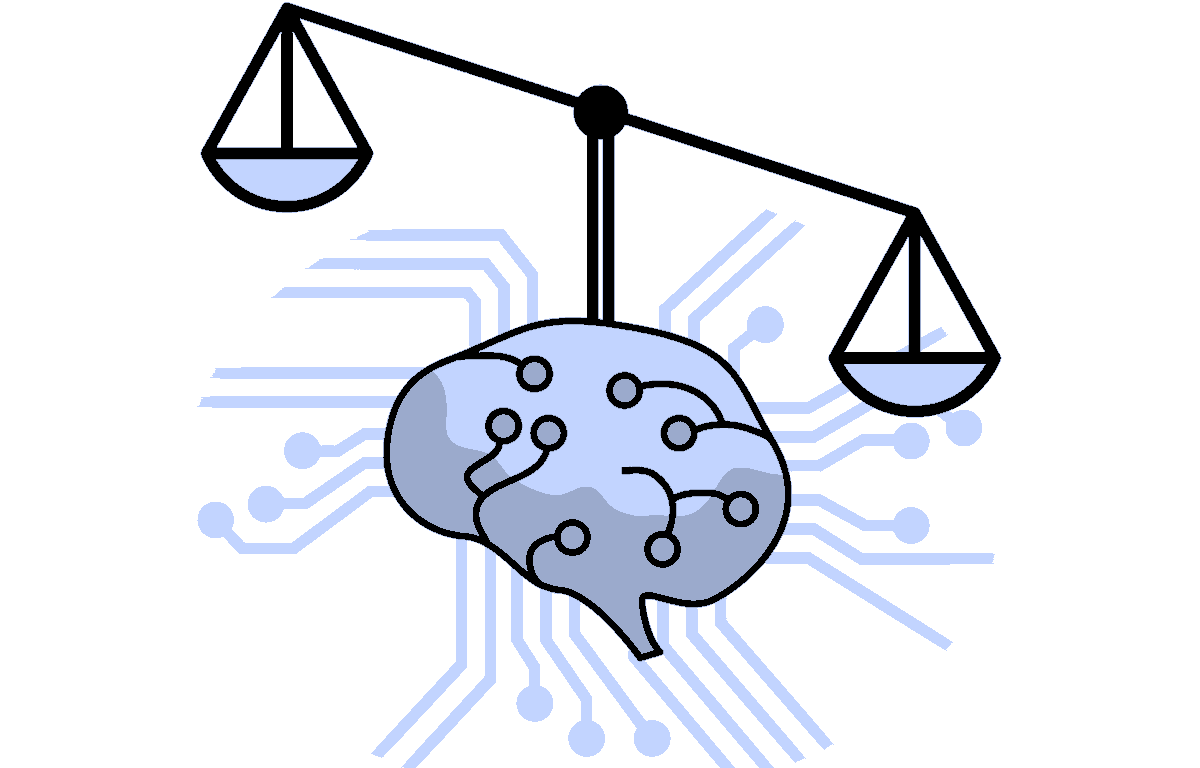
Our Principles
-
Data alone should never drive policy.
We want to understand what you, our visitors, think about "unfair fairness" and we believe that these perspectives are important — but we also believe that they are in no way the whole story.
-
There are multiple applications to consider.
We know that "unfair fairness" can manifest in different contexts, creating different types of harm. That's why our games explore two very different settings with different consequences.
-
Education before experimentation.
As much as we want to learn from you, we want you to learn from us first. That's why our games are educational tools first and foremost, with the option to participate in surveys after viewing educational content.
The Research
Learn more about the research behind Unfair Fairness.
Based at the University of Oxford's Oxford Internet Institute, Professors Brent Mittelstadt, Sandra Wachter, and Chris Russell have led the effort to popularize "unfair fairness" and the harms associated with attempting to achieve "fair" algortihms via strict egalitarianism. This research — alongside a growing body of literature — inspired our work to raise awareness about these issues.
Play Our Educational Games
See how "unfair fairness" may manifest in the real world.